How the AI boom will change the rules of enterprise data centre hardware asset management
AI brings both challenges and solutions to data centre hardware management. The most successful operators will be those who apply AI to develop innovative solutions to the capacity and environmental challenges this new world presents.
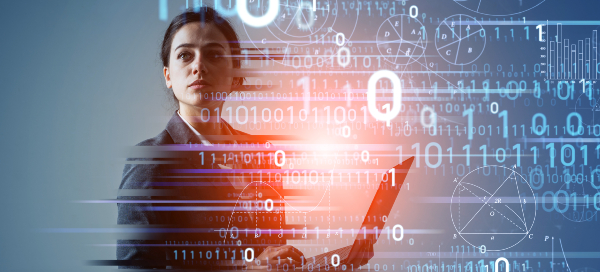
The AI boom is both an opportunity and a challenge for enterprise data centres. AI promises to simplify some of the most challenging management tasks but it will also require infrastructure upgrades that tax existing power and cooling systems and bring more workload variability.
Let’s first look at the benefits
Hardware asset management in data centres has traditionally been an error-prone manual process involving tedious tracking, evaluation, and maintenance of physical and digital assets.
AI can automate much of this work while also enhancing accuracy and driving higher efficiencies.
AI-driven tools can use sensors and software agents to track inventory, monitor equipment status, and check for compliance with policies and regulations.
AI can also enable the game-changing practice of predictive maintenance.
Traditionally, data centre maintenance has been primarily reactive, addressing issues only when failures occurred or were imminent.
AI algorithms can analyse vast quantities of sensor data from IT equipment, power systems, and cooling units to identify subtle shifts in performance metrics that indicate the likelihood of equipment failure. Data centre personnel can repair or replace equipment during scheduled downtime rather than fighting fires. This proactive approach can drastically reduce both downtime and unnecessary maintenance on assets that are in good working condition.
Optimising space and energy
AI can also play a pivotal role in asset utilisation, one of the most complex tasks facing data centre managers. Equipment must be run at high performance levels to optimise power and floor space and minimise the energy wasted by running and cooling idle systems. Sensors can detect when servers are unused or underpowered, and AI automation can adjust virtualisation levels to reduce waste.
AI helps optimise electricity usage by analysing real-time power draw, temperature, and workload distribution data. Algorithms can then dynamically adjust cooling systems, power delivery, and server workloads to achieve peak efficiency. This reduces operational costs and extends equipment life since environmental factors are one of the leading causes of equipment failure.
Data centre capacity planning is a delicate balancing act that requires sufficient resources to meet current and future demands without overprovisioning. AI can analyse historical data, workload trends, and business forecasts to predict future resource requirements with precision humans can’t match. This avoids costly overspending on unused resources while ensuring enough capacity for future growth.
There are also indications that AI algorithms can achieve upwards of 90% accuracy in identifying components for recycling, while reducing health risks with robotic sorting. Given that less than 20% of e-waste is recycled today, this capability could make e-waste recycling faster and more affordable.
Despite all these benefits, integrating AI into existing on-premises data centre infrastructure is not without its asset management challenges. The need for substantial investments in AI technology and the skilled personnel to manage AI-driven systems are significant hurdles that enterprises must navigate if they choose to host their own AI workloads.
AI challenges
While most AI model training currently occurs in the cloud, AI applications are expected to trigger renewed investments in on-premises infrastructure. Reasons include data residency requirements, latency, the cost of transferring large data sets back and forth to cloud services and privacy/security regulations.
A recent Nutanix survey of IT decision-makers found that
- 92%
expect IT costs to increase due to AI applications and services
- 85%
plan to increase investments in modernising IT infrastructure to support AI workloads
- Nearly 60%
expect to run AI inferencing workloads – which apply the models to real-world use cases - to reside in their own data centres or private clouds.
As AI adoption grows, so will the demand for data centre capacity. Training AI models requires clusters of graphic processing units or tensor processing units working in parallel. These transistor-heavy chips require 10 to 15 times the energy of a traditional CPU. Many generative AI models also have billions of parameters and that can stretch training times to months. AI processing is expected to drive much of the growth in data centre construction in the coming years. If generative AI boosts the data centre compound annual growth rate to 25%, overall capacity will need to triple in the next five years.
These factors will continue to increase the need for ever-denser racks in the data centre. High-performance computing environments, which are increasingly common in AI scenarios, may demand up to 50 kilowatts.
Increased performance specs will speed up the already rapid pace of equipment upgrades and hardware refreshes, making it more important than ever to ensure that decommissioned equipment is adapted to other uses, resold or responsibly recycled to minimise environmental impact. Data centre operators will need to step up their focus on the circularity principles of reuse, refurbishment and responsible disposal.
Careful planning will be required to accommodate the increased power, cooling, and physical space requirements of AI. This means a heightened focus on strategic asset management for enterprise data centre operators to ensure timely and cost-effective buildouts or retrofits – or a shift to a colocation strategy.
AI brings both challenges and solutions to data centre hardware management. The most successful operators will be those who apply AI to develop innovative solutions to the capacity and environmental challenges this new world presents.
Related resources
View More Resources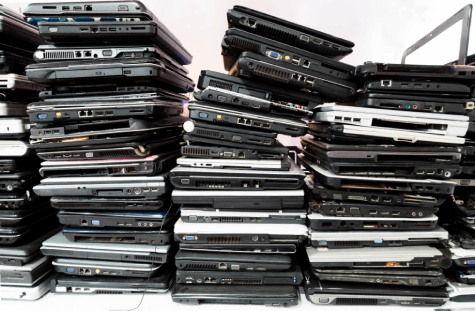